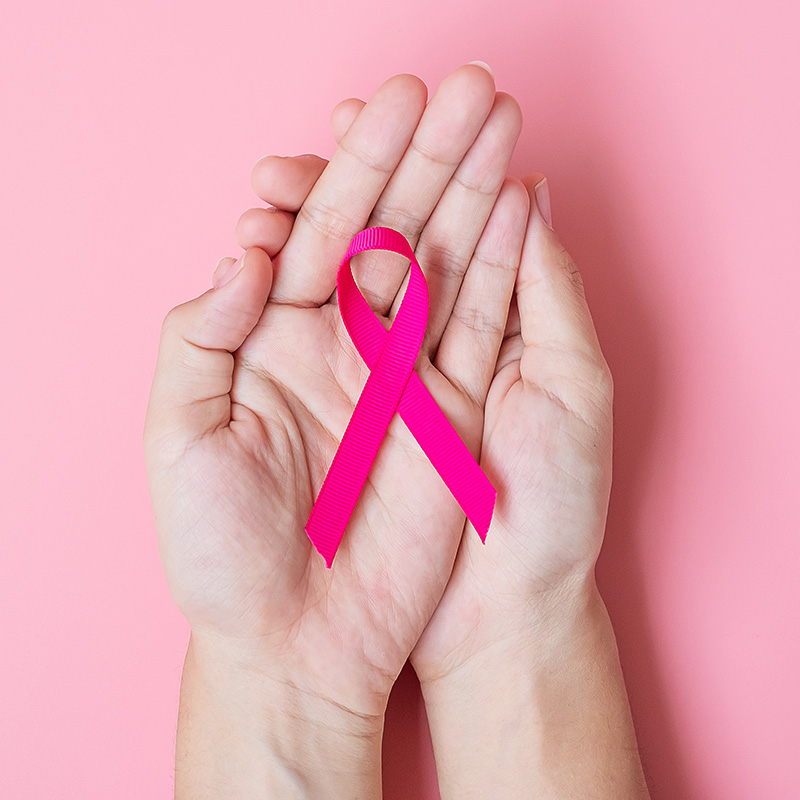
By: Su K.
Year: 2021
School: Capistrano Valley High
Grade: 11
Science Teacher: Cheryl Johnson
Abstract
About 1 in 8 U.S. women will develop breast cancer in their lifetime. Breast density is a strong indicator for breast cancer. Women with extremely dense breasts have a sixfold greater risk of developing breast cancer. This study is about the assessment of breast density by using unsupervised deep learning algorithms. I trained a variational auto-encoder algorithm on 6,987 patient mammograms without any manual annotations of the dense regions of the breast. With the use of the encoder model, I was able to predict the breast density as the ratio of the fibro glandular tissue to the whole breast accurately.
Analysis and Results
Pearson correlation between the mean of masked latent and the original FGT/breast ratio was calculated as 0.68. Linear regression showed a mean absolute error of 0.05. The breast density (FGT/breast ratio) shown as the output y can be calculated by using the formula below, where the input x stands for the mean of masked latent: y = 0.23x + 0.38
Even though decoder predictions show more clear distinction between FGT and breast in lower number of features, somehow higher latent dimensions result in higher correlation. The model must be learning more about contrast in those additional features.
The data supports my hypothesis that an unsupervised deep learning algorithm such as VAE can be used to predict breast density. Even though supervised algorithms such as regular CNN and U-Net models still provide higher accuracy, radiologists need to spend a long time to annotate MRIs, CT scans, and mammograms manually. Unsupervised techniques will be the next breakthrough in the use of AI in medical diagnosis as there will be no need to annotate images anymore. This study proves that new unsupervised techniques can be used to address some of the current medical diagnosis needs.