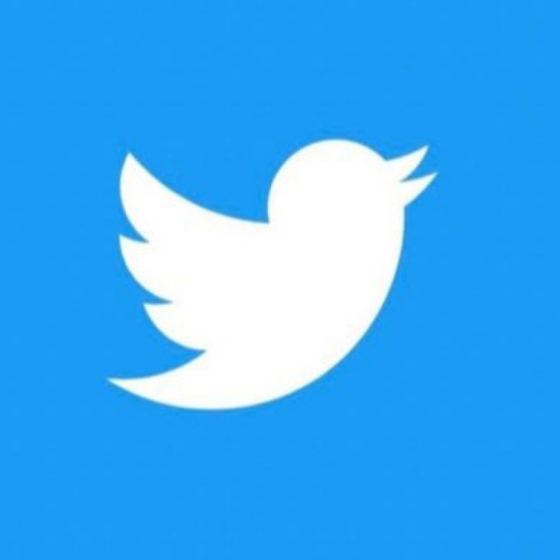
By: Marissa H.
Year: 2023
School: Woodbridge High
Grade: 9
Science Teacher: Tracy Walla
In a world where major tragedies seem to occur all too frequently, one pressing concern is the dwindling public attention span that follows these events. The window of opportunity to implement meaningful changes with substantial public support in the aftermath of such tragedies has been shrinking. This is particularly evident in the aftermath of events like deadly hurricanes and fatal shootings, which may initially capture the public’s interest but quickly fade into obscurity or get overshadowed by other news. This project, led by 9th grader Marissa, delves into this issue by examining changes in gun-related sentiment on Twitter following six major mass shootings. The aim is to determine if there exists an optimal window of opportunity for action for either side of the gun policy debate.
To accomplish this, Marissa embarked on a data-driven journey. She collected two million tweets related to six major mass shootings, comprising three non-school shootings and three school-related shootings. From this vast dataset, she randomly sampled 6,000 tweets, which were then meticulously hand-labeled into three categories: neutral, anti-gun, and pro-gun. These labeled tweets were further categorized into non-school and school-related subsets.
Marissa employed three different machine learning models – the Random Forest Classifier, Multinomial Naive Bayes, and Support Vector Machine – to classify the two subsets of tweets. The model with the highest accuracy, the Random Forest Classifier, was selected to classify all two million tweets, enabling a comprehensive analysis of public sentiment.
The results of this project provided insightful and thought-provoking conclusions. First and foremost, both of the initial hypotheses were supported by the data. Anti-gun sentiment saw a significant spike immediately after both school and non-school shootings, but this sentiment began to decline sharply within the next 12 to 22 days. On the other hand, pro-gun sentiment also experienced fluctuations but with less volatility.
Interestingly, the study revealed a distinction between school and non-school shootings. Non-school shootings generally yielded more neutral and pro-gun sentiment, while school shootings were associated with a stronger anti-gun sentiment and a more subdued pro-gun response. This distinction suggests that school shootings may evoke a more emotional and polarized response from the public.
The findings suggest that to maximize supportive sentiment for gun-related policy changes, actions should be strategically timed. Anti-gun actions appear to be most effective when pursued within the first two weeks following a shooting event, while pro-gun sentiment appears to remain relatively constant over time. This nuanced understanding of the timing of public sentiment can be instrumental for lawmakers, lobbyists, NGOs, and other stakeholders seeking to optimize public support for new policies related to gun control.