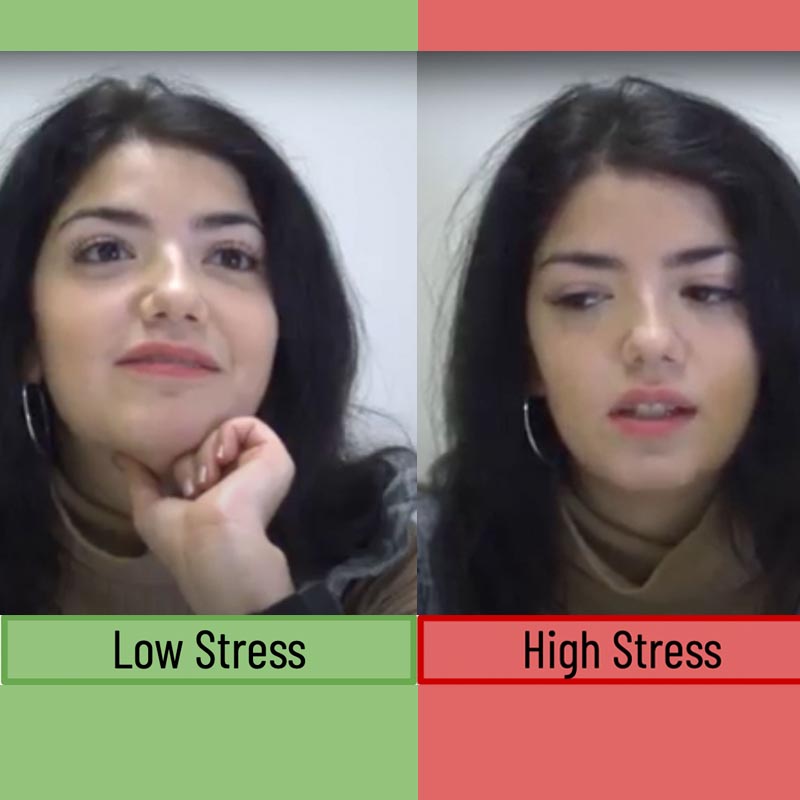
By: Akshata T.
Year: 2022
School: Aliso Niguel High School
Grade: 11
Science Teacher: Robert Jansen
Stress detection through video is a fundamentally important concept. As autonomous vehicles become more commonplace and human alertness subsides, instantaneous detection of physiological features has a prominent role in ensuring human safety in dire situations. Autopilot or other safety mechanisms can be enabled in case of severe stress experienced by drivers or pilots.
Akshata’s project incorporates the newest advancements in various domains of artificial intelligence to bring about end-to-end, non-invasive, real time detection of stress through video. This project uses several metrics to perform holistic detection of a user’s stress level. It finds the user’s heart rate through video by processing minute changes in skin coloration. The model also detects emotional cues and other movements through recordings. Further, it uses a series of 3D convolution networks to learn new features that correspond with stress. By integrating each of these measurements, Akshata’s project provides accurate, contactless detection of stress in real-time. The materials used were a Windows computer and Google Collaboratory notebooks.
“My project incorporates the newest advancements in artificial intelligence to bring about end-to-end, non-invasive detection of stress through video,” Akshata explains. “This project uses several metrics to perform holistic detection of a user’s stress level. It finds the user’s heart rate through video by processing changes in skin coloration through Spatio-temporal neural networks. It uses machine learning to detect emotional expressions through facial recordings. Finally, eyebrow and lip movement are analyzed. I trained the models on data from two publicly available datasets, PURE and CK+, obtained after signing agreements with providers.”
“My project’s emotion detection module had an accuracy of 85%, nearing state-of-the-art systems. The remote heart rate detection achieved a mean absolute waveform error of 1.1014. By integrating these three measurements, my project provides accurate, contactless detection of stress through video.”
The Emotion Recognition model, after training on 100 epochs, resulted in a testing accuracy of 85%. This outperforms the results achieved in the study of Song (“Facial Expression Emotion Recognition Model Integrating Philosophy and Machine Learning Theory”) and others.
The Heart rate detection model (Spatio-Temporal Neural Network) resulted in a mean absolute waveform error of 1.1014. The lighting conditions and sequence length (frames in each video grouping) affected the model’s output.
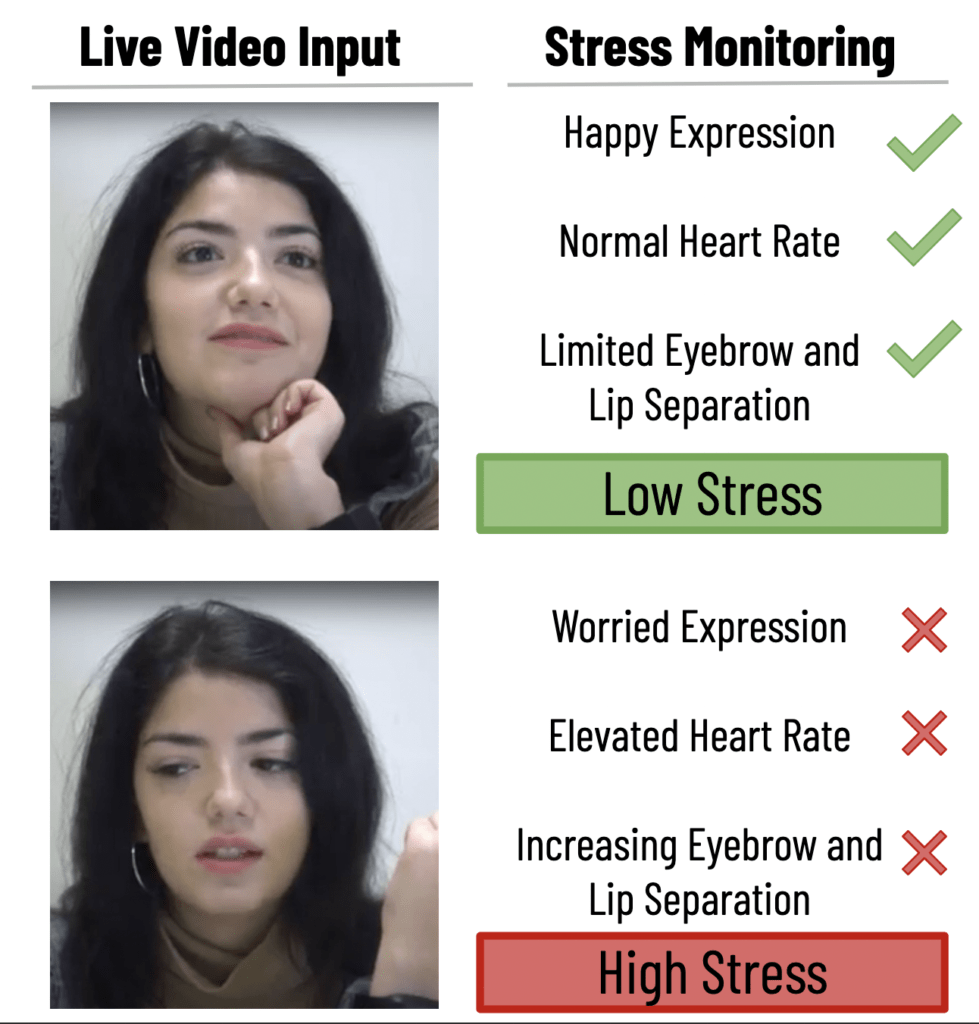